We were delighted to host American statistician Janet Wittes in Hamilton this week as our 5th Annual Janice Pogue Lectureship on Biostatistics, organized by PHRI’s Statistics Department.
Wittes spoke at our Hamilton office and was streamed live in a Zoom webinar. Well-known for her research on the design of clinical trials, Wittes completed her Ph.D. in Statistics at Harvard University in 1970, and after serving in several academic positions, she joined the National Heart Lung and Blood Institute’s Biostatistics Research Branch as its Chief in 1983. In 1990 she founded her consulting firm WCG Statistics Collaborative in Washington, DC, and is currently its President Emerita.
In her Janice Pogue Lectureship, Interim Analyses: Rules or Guidelines? (download slides) which she subtitled “a guide from and for the perplexed.” Wittes describes the challenge as follows:
“Those of us involved in randomized controlled trials – especially trials that test the effect of an intervention on a hard clinical outcome – are conversant with formal interim analyses permitting a DSMB to recommend stopping a trial if there is little hope that the experimental intervention will show convincing evidence of benefit (a.k.a. futility) or if the data show ‘overwhelming’ evidence of benefit.”
However, she added, “hidden behind the word “overwhelming’ lurks the need to protect the Type I error rate whether one formulates the trial in a frequentist or Bayesian manner… Sometimes, however, the data from a trial do not obey what the designers anticipated. A boundary may be crossed allowing a formal declaration of benefit, but the DSMB is hesitant to recommend stopping because it fears that the trial has still not answered important questions… In other cases, the data show extremely strong evidence of benefit, but the trajectory of the observed trend has not crossed a boundary.”
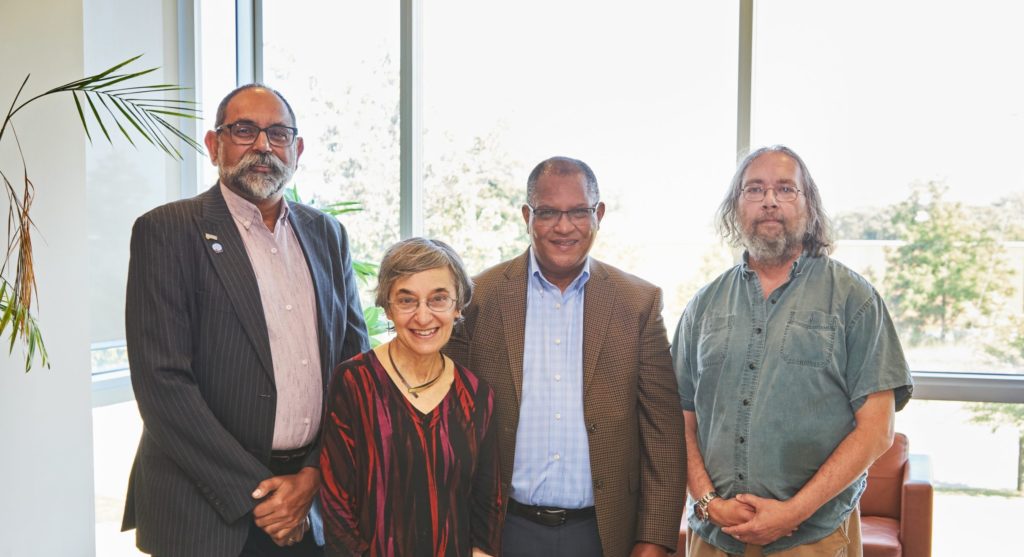
Left to right: PHRI Statistics Director Shrikant Bangdiwala; guest lecturer Janet Wittes; Lehana Thabane, VP, Research, St. Joseph’s Healthcare Hamilton; and Pogue’s widower David White at the 5th annual Janice Pogue Lectureship in Biostatistics held June 28, 2022, at PHRI’s office.
Wittes used real-world examples, including two from studies performed by PHRI, to address both problems of: crossing a boundary that allows a formal declaration of efficacy but not wanting to stop; and not crossing a boundary, but feeling the data are so strong that continuing is unscientific.
(Shout out to Tina Guagliano, Administrative Assistant, Statistics, for her calm efficiency in single-handedly organizing the Pogue Lectureship!)
Once in Love with Hazard Ratios
While in Hamilton, Wittes also gave a talk at the Health Evidence and Impact (HEI) department at McMaster University, on the topic, “Once in love with hazard ratios.” She describes the talk, in part:
“Cox’s proportional hazards model (1972) gave us the tool we were looking for: we could now calculate something called the hazard ratio (HR) which summarized in one number the effect of an experimental treatment relative to control over the entire period covered by the Kaplan-Meier curves.
We had everything we needed – a visual representation of survival over time, a way of testing the difference in the curves at specific time, a method for assigning a p-value to assess the degree to which the difference between the curves was inconsistent with chance, and a summary statistic, the HR, to describe the magnitude of the difference between curves.
We knew that the log-rank test was optimal when the curves had proportional hazards, but valid even when they did not. The Cox model, on the other hand, required proportional hazards, but if the hazards were not far from proportionality, the model was good enough to use.
We invented sloppy language to describe what a HR was, suspecting that many non-statisticians would not understand the technical language of “hazard”. This talk addresses what a HR really is, how it applies in the case of non-proportional hazards, and asks whether we should be summarizing estimates from survival curves with other statistics – e.g., perhaps back to comparison of events at specific times or the increasingly popular restricted mean survival time.”
Download Wittes’ slides, Once in Love with Hazard Ratios (PDF).